Ensuring performance of sketching/streaming algorithm (countSketch) The 2019 Stack Overflow Developer Survey Results Are In Announcing the arrival of Valued Associate #679: Cesar Manara Planned maintenance scheduled April 17/18, 2019 at 00:00UTC (8:00pm US/Eastern)Implementing the CutHill-McKee AlgorithmStreaming variable length integers from a fileStreaming CollatzChanging algorithm to avoid looping with iterrowsStreaming int supportStreaming learning OCamlSingle-pass clustering algorithm for sparse matricesOptimization of algorithm performanceOptimizing priority queue streaming algorithm in C++Templated byte streaming
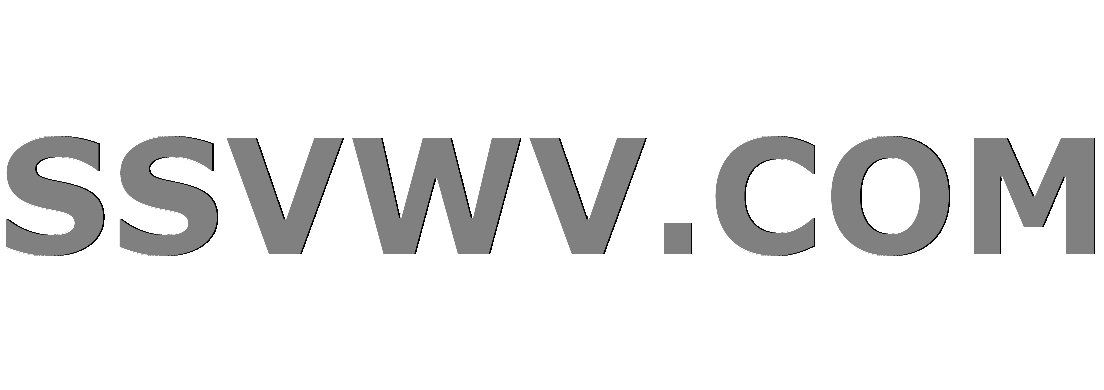
Multi tool use
Mortgage adviser recommends a longer term than necessary combined with overpayments
Make it rain characters
Variable with quotation marks "$()"
Is every episode of "Where are my Pants?" identical?
Student Loan from years ago pops up and is taking my salary
How do you keep chess fun when your opponent constantly beats you?
Circular reasoning in L'Hopital's rule
Would an alien lifeform be able to achieve space travel if lacking in vision?
What can I do if neighbor is blocking my solar panels intentionally?
Homework question about an engine pulling a train
Can the Right Ascension and Argument of Perigee of a spacecraft's orbit keep varying by themselves with time?
Huge performance difference of the command find with and without using %M option to show permissions
how can a perfect fourth interval be considered either consonant or dissonant?
Is it ok to offer lower paid work as a trial period before negotiating for a full-time job?
One-dimensional Japanese puzzle
Why doesn't a hydraulic lever violate conservation of energy?
What aspect of planet Earth must be changed to prevent the industrial revolution?
If I score a critical hit on an 18 or higher, what are my chances of getting a critical hit if I roll 3d20?
should truth entail possible truth
Didn't get enough time to take a Coding Test - what to do now?
ELI5: Why do they say that Israel would have been the fourth country to land a spacecraft on the Moon and why do they call it low cost?
Working through the single responsibility principle (SRP) in Python when calls are expensive
How to read αἱμύλιος or when to aspirate
Are spiders unable to hurt humans, especially very small spiders?
Ensuring performance of sketching/streaming algorithm (countSketch)
The 2019 Stack Overflow Developer Survey Results Are In
Announcing the arrival of Valued Associate #679: Cesar Manara
Planned maintenance scheduled April 17/18, 2019 at 00:00UTC (8:00pm US/Eastern)Implementing the CutHill-McKee AlgorithmStreaming variable length integers from a fileStreaming CollatzChanging algorithm to avoid looping with iterrowsStreaming int supportStreaming learning OCamlSingle-pass clustering algorithm for sparse matricesOptimization of algorithm performanceOptimizing priority queue streaming algorithm in C++Templated byte streaming
.everyoneloves__top-leaderboard:empty,.everyoneloves__mid-leaderboard:empty,.everyoneloves__bot-mid-leaderboard:empty margin-bottom:0;
$begingroup$
I have implemented what is know as a countSketch in python (page 17: https://arxiv.org/pdf/1411.4357.pdf) but my implementation is currently lacking in performance. The algorithm is to compute the product SA
where A
is an n x d
matrix, S
is m x n
matrix defined as follows: for every column of S
uniformly at random select a row (hash bucket) from the m
rows and for that given row, uniformly at random select +1 or -1. So S is a matrix with exactly one nonzero in every column and otherwise all zero.
My intention is to compute SA
in a streaming fashion by reading the entries of A
. The idea for my implementation is as follows: observe a sequence of triples (i,j,A_ij)
and return a sequence (h(i), j, s(i)A_ij)
where:
- h(i)
is a hash bucket (row of matrix chosen uniformly at random from the m
possible rows of S
- s(i)
is the random sign function as described above.
I have assumed that the matrix is in row order so that the first row of A
arrives in its entirety before the next row of A
arrives because this limits the number of calls I need to select a random bucket or the need to use a hash library. I have also assumed that the number of nonzero entries (or the length of the input stream) is known so that I can efficiently encode the iteration.
My problem is that the matrix should compute (1+error)*||Ax||^2 <= ||SAx||^2 <= (1+error)*||Ax||^2
and also have the difference in frobenius norms between A^T S^T S A
and A^T A
being small. However, while my implementation for the first condition seems to be true, the latter is consistently too small. I was wondering if there is an obvious reason for this that I am missing because it seems to be underestimating the latter quantity.
I am open to feedback on changing the code if there are obvious improvements to be made.
nb. If you don't want to run using numba
then just comment out the import and the function decorator and it will run in standard numpy/scipy.
import numpy as np
import numpy.random as npr
import scipy.sparse as sparse
from scipy.sparse import coo_matrix
import numba
from numba import jit
@jit(nopython=True) # comment this if want just numpy
def countSketch(input_rows, input_data,
input_nnz,
sketch_size, seed=None):
'''
input_rows: row indices for data (can be repeats)
input_data: values seen in row location,
input_nnz : number of nonzers in the data (can replace with
len(input_data) but avoided here for speed)
sketch_size: int
seed=None : random seed
'''
hashed_rows = np.empty(input_rows.shape,dtype=np.int32)
current_row = 0
hash_val = npr.choice(sketch_size)
sign_val = npr.choice(np.array([-1.0,1.0]))
#print(hash_val)
hashed_rows[0] = hash_val
#print(hash_val)
for idx in np.arange(input_nnz):
print(idx)
row_id = input_rows[idx]
data_val = input_data[idx]
if row_id == current_row:
hashed_rows[idx] = hash_val
input_data[idx] = sign_val*data_val
else:
# make new hashes
hash_val = npr.choice(sketch_size)
sign_val = npr.choice(np.array([-1.0,1.0]))
hashed_rows[idx] = hash_val
input_data[idx] = sign_val*data_val
return hashed_rows, input_data
def sort_row_order(input_data):
sorted_row_column = np.array((input_data.row,
input_data.col,
input_data.data))
idx = np.argsort(sorted_row_column[0])
sorted_rows = np.array(sorted_row_column[0,idx], dtype=np.int32)
sorted_cols = np.array(sorted_row_column[1,idx], dtype=np.int32)
sorted_data = np.array(sorted_row_column[2,idx], dtype=np.float64)
return sorted_rows, sorted_cols, sorted_data
if __name__=="__main__":
import time
from tabulate import tabulate
matrix = sparse.random(1000, 50, 0.1)
x = np.random.randn(matrix.shape[1])
true_norm = np.linalg.norm(matrix@x,ord=2)**2
tidy_data = sort_row_order(matrix)
sketch_size = 300
start = time.time()
hashed_rows, sketched_data = countSketch(tidy_data[0],
tidy_data[2], matrix.nnz,sketch_size)
duration_slow = time.time() - start
S_A = sparse.coo_matrix((sketched_data, (hashed_rows,matrix.col)))
approx_norm_slow = np.linalg.norm(S_A@x,ord=2)**2
rel_error_slow = approx_norm_slow/true_norm
#print("Sketch time: ".format(duration_slow))
start = time.time()
hashed_rows, sketched_data = countSketch(tidy_data[0],
tidy_data[2], matrix.nnz,sketch_size)
duration = time.time() - start
#print("Sketch time: ".format(duration))
S_A = sparse.coo_matrix((sketched_data, (hashed_rows,matrix.col)))
approx_norm = np.linalg.norm(S_A@x,ord=2)**2
rel_error = approx_norm/true_norm
#print("Relative norms: ".format(approx_norm/true_norm))
print(tabulate([[duration_slow, rel_error_slow, 'Yes'],
[duration, rel_error, 'No']],
headers=['Sketch Time', 'Relative Error', 'Dry Run'],
tablefmt='orgtbl'))
python algorithm numpy stream scipy
$endgroup$
bumped to the homepage by Community♦ 14 mins ago
This question has answers that may be good or bad; the system has marked it active so that they can be reviewed.
add a comment |
$begingroup$
I have implemented what is know as a countSketch in python (page 17: https://arxiv.org/pdf/1411.4357.pdf) but my implementation is currently lacking in performance. The algorithm is to compute the product SA
where A
is an n x d
matrix, S
is m x n
matrix defined as follows: for every column of S
uniformly at random select a row (hash bucket) from the m
rows and for that given row, uniformly at random select +1 or -1. So S is a matrix with exactly one nonzero in every column and otherwise all zero.
My intention is to compute SA
in a streaming fashion by reading the entries of A
. The idea for my implementation is as follows: observe a sequence of triples (i,j,A_ij)
and return a sequence (h(i), j, s(i)A_ij)
where:
- h(i)
is a hash bucket (row of matrix chosen uniformly at random from the m
possible rows of S
- s(i)
is the random sign function as described above.
I have assumed that the matrix is in row order so that the first row of A
arrives in its entirety before the next row of A
arrives because this limits the number of calls I need to select a random bucket or the need to use a hash library. I have also assumed that the number of nonzero entries (or the length of the input stream) is known so that I can efficiently encode the iteration.
My problem is that the matrix should compute (1+error)*||Ax||^2 <= ||SAx||^2 <= (1+error)*||Ax||^2
and also have the difference in frobenius norms between A^T S^T S A
and A^T A
being small. However, while my implementation for the first condition seems to be true, the latter is consistently too small. I was wondering if there is an obvious reason for this that I am missing because it seems to be underestimating the latter quantity.
I am open to feedback on changing the code if there are obvious improvements to be made.
nb. If you don't want to run using numba
then just comment out the import and the function decorator and it will run in standard numpy/scipy.
import numpy as np
import numpy.random as npr
import scipy.sparse as sparse
from scipy.sparse import coo_matrix
import numba
from numba import jit
@jit(nopython=True) # comment this if want just numpy
def countSketch(input_rows, input_data,
input_nnz,
sketch_size, seed=None):
'''
input_rows: row indices for data (can be repeats)
input_data: values seen in row location,
input_nnz : number of nonzers in the data (can replace with
len(input_data) but avoided here for speed)
sketch_size: int
seed=None : random seed
'''
hashed_rows = np.empty(input_rows.shape,dtype=np.int32)
current_row = 0
hash_val = npr.choice(sketch_size)
sign_val = npr.choice(np.array([-1.0,1.0]))
#print(hash_val)
hashed_rows[0] = hash_val
#print(hash_val)
for idx in np.arange(input_nnz):
print(idx)
row_id = input_rows[idx]
data_val = input_data[idx]
if row_id == current_row:
hashed_rows[idx] = hash_val
input_data[idx] = sign_val*data_val
else:
# make new hashes
hash_val = npr.choice(sketch_size)
sign_val = npr.choice(np.array([-1.0,1.0]))
hashed_rows[idx] = hash_val
input_data[idx] = sign_val*data_val
return hashed_rows, input_data
def sort_row_order(input_data):
sorted_row_column = np.array((input_data.row,
input_data.col,
input_data.data))
idx = np.argsort(sorted_row_column[0])
sorted_rows = np.array(sorted_row_column[0,idx], dtype=np.int32)
sorted_cols = np.array(sorted_row_column[1,idx], dtype=np.int32)
sorted_data = np.array(sorted_row_column[2,idx], dtype=np.float64)
return sorted_rows, sorted_cols, sorted_data
if __name__=="__main__":
import time
from tabulate import tabulate
matrix = sparse.random(1000, 50, 0.1)
x = np.random.randn(matrix.shape[1])
true_norm = np.linalg.norm(matrix@x,ord=2)**2
tidy_data = sort_row_order(matrix)
sketch_size = 300
start = time.time()
hashed_rows, sketched_data = countSketch(tidy_data[0],
tidy_data[2], matrix.nnz,sketch_size)
duration_slow = time.time() - start
S_A = sparse.coo_matrix((sketched_data, (hashed_rows,matrix.col)))
approx_norm_slow = np.linalg.norm(S_A@x,ord=2)**2
rel_error_slow = approx_norm_slow/true_norm
#print("Sketch time: ".format(duration_slow))
start = time.time()
hashed_rows, sketched_data = countSketch(tidy_data[0],
tidy_data[2], matrix.nnz,sketch_size)
duration = time.time() - start
#print("Sketch time: ".format(duration))
S_A = sparse.coo_matrix((sketched_data, (hashed_rows,matrix.col)))
approx_norm = np.linalg.norm(S_A@x,ord=2)**2
rel_error = approx_norm/true_norm
#print("Relative norms: ".format(approx_norm/true_norm))
print(tabulate([[duration_slow, rel_error_slow, 'Yes'],
[duration, rel_error, 'No']],
headers=['Sketch Time', 'Relative Error', 'Dry Run'],
tablefmt='orgtbl'))
python algorithm numpy stream scipy
$endgroup$
bumped to the homepage by Community♦ 14 mins ago
This question has answers that may be good or bad; the system has marked it active so that they can be reviewed.
$begingroup$
"... the latter is consistently too small_" does that mean it is not working correctly?
$endgroup$
– Sᴀᴍ Onᴇᴌᴀ
May 17 '18 at 21:46
add a comment |
$begingroup$
I have implemented what is know as a countSketch in python (page 17: https://arxiv.org/pdf/1411.4357.pdf) but my implementation is currently lacking in performance. The algorithm is to compute the product SA
where A
is an n x d
matrix, S
is m x n
matrix defined as follows: for every column of S
uniformly at random select a row (hash bucket) from the m
rows and for that given row, uniformly at random select +1 or -1. So S is a matrix with exactly one nonzero in every column and otherwise all zero.
My intention is to compute SA
in a streaming fashion by reading the entries of A
. The idea for my implementation is as follows: observe a sequence of triples (i,j,A_ij)
and return a sequence (h(i), j, s(i)A_ij)
where:
- h(i)
is a hash bucket (row of matrix chosen uniformly at random from the m
possible rows of S
- s(i)
is the random sign function as described above.
I have assumed that the matrix is in row order so that the first row of A
arrives in its entirety before the next row of A
arrives because this limits the number of calls I need to select a random bucket or the need to use a hash library. I have also assumed that the number of nonzero entries (or the length of the input stream) is known so that I can efficiently encode the iteration.
My problem is that the matrix should compute (1+error)*||Ax||^2 <= ||SAx||^2 <= (1+error)*||Ax||^2
and also have the difference in frobenius norms between A^T S^T S A
and A^T A
being small. However, while my implementation for the first condition seems to be true, the latter is consistently too small. I was wondering if there is an obvious reason for this that I am missing because it seems to be underestimating the latter quantity.
I am open to feedback on changing the code if there are obvious improvements to be made.
nb. If you don't want to run using numba
then just comment out the import and the function decorator and it will run in standard numpy/scipy.
import numpy as np
import numpy.random as npr
import scipy.sparse as sparse
from scipy.sparse import coo_matrix
import numba
from numba import jit
@jit(nopython=True) # comment this if want just numpy
def countSketch(input_rows, input_data,
input_nnz,
sketch_size, seed=None):
'''
input_rows: row indices for data (can be repeats)
input_data: values seen in row location,
input_nnz : number of nonzers in the data (can replace with
len(input_data) but avoided here for speed)
sketch_size: int
seed=None : random seed
'''
hashed_rows = np.empty(input_rows.shape,dtype=np.int32)
current_row = 0
hash_val = npr.choice(sketch_size)
sign_val = npr.choice(np.array([-1.0,1.0]))
#print(hash_val)
hashed_rows[0] = hash_val
#print(hash_val)
for idx in np.arange(input_nnz):
print(idx)
row_id = input_rows[idx]
data_val = input_data[idx]
if row_id == current_row:
hashed_rows[idx] = hash_val
input_data[idx] = sign_val*data_val
else:
# make new hashes
hash_val = npr.choice(sketch_size)
sign_val = npr.choice(np.array([-1.0,1.0]))
hashed_rows[idx] = hash_val
input_data[idx] = sign_val*data_val
return hashed_rows, input_data
def sort_row_order(input_data):
sorted_row_column = np.array((input_data.row,
input_data.col,
input_data.data))
idx = np.argsort(sorted_row_column[0])
sorted_rows = np.array(sorted_row_column[0,idx], dtype=np.int32)
sorted_cols = np.array(sorted_row_column[1,idx], dtype=np.int32)
sorted_data = np.array(sorted_row_column[2,idx], dtype=np.float64)
return sorted_rows, sorted_cols, sorted_data
if __name__=="__main__":
import time
from tabulate import tabulate
matrix = sparse.random(1000, 50, 0.1)
x = np.random.randn(matrix.shape[1])
true_norm = np.linalg.norm(matrix@x,ord=2)**2
tidy_data = sort_row_order(matrix)
sketch_size = 300
start = time.time()
hashed_rows, sketched_data = countSketch(tidy_data[0],
tidy_data[2], matrix.nnz,sketch_size)
duration_slow = time.time() - start
S_A = sparse.coo_matrix((sketched_data, (hashed_rows,matrix.col)))
approx_norm_slow = np.linalg.norm(S_A@x,ord=2)**2
rel_error_slow = approx_norm_slow/true_norm
#print("Sketch time: ".format(duration_slow))
start = time.time()
hashed_rows, sketched_data = countSketch(tidy_data[0],
tidy_data[2], matrix.nnz,sketch_size)
duration = time.time() - start
#print("Sketch time: ".format(duration))
S_A = sparse.coo_matrix((sketched_data, (hashed_rows,matrix.col)))
approx_norm = np.linalg.norm(S_A@x,ord=2)**2
rel_error = approx_norm/true_norm
#print("Relative norms: ".format(approx_norm/true_norm))
print(tabulate([[duration_slow, rel_error_slow, 'Yes'],
[duration, rel_error, 'No']],
headers=['Sketch Time', 'Relative Error', 'Dry Run'],
tablefmt='orgtbl'))
python algorithm numpy stream scipy
$endgroup$
I have implemented what is know as a countSketch in python (page 17: https://arxiv.org/pdf/1411.4357.pdf) but my implementation is currently lacking in performance. The algorithm is to compute the product SA
where A
is an n x d
matrix, S
is m x n
matrix defined as follows: for every column of S
uniformly at random select a row (hash bucket) from the m
rows and for that given row, uniformly at random select +1 or -1. So S is a matrix with exactly one nonzero in every column and otherwise all zero.
My intention is to compute SA
in a streaming fashion by reading the entries of A
. The idea for my implementation is as follows: observe a sequence of triples (i,j,A_ij)
and return a sequence (h(i), j, s(i)A_ij)
where:
- h(i)
is a hash bucket (row of matrix chosen uniformly at random from the m
possible rows of S
- s(i)
is the random sign function as described above.
I have assumed that the matrix is in row order so that the first row of A
arrives in its entirety before the next row of A
arrives because this limits the number of calls I need to select a random bucket or the need to use a hash library. I have also assumed that the number of nonzero entries (or the length of the input stream) is known so that I can efficiently encode the iteration.
My problem is that the matrix should compute (1+error)*||Ax||^2 <= ||SAx||^2 <= (1+error)*||Ax||^2
and also have the difference in frobenius norms between A^T S^T S A
and A^T A
being small. However, while my implementation for the first condition seems to be true, the latter is consistently too small. I was wondering if there is an obvious reason for this that I am missing because it seems to be underestimating the latter quantity.
I am open to feedback on changing the code if there are obvious improvements to be made.
nb. If you don't want to run using numba
then just comment out the import and the function decorator and it will run in standard numpy/scipy.
import numpy as np
import numpy.random as npr
import scipy.sparse as sparse
from scipy.sparse import coo_matrix
import numba
from numba import jit
@jit(nopython=True) # comment this if want just numpy
def countSketch(input_rows, input_data,
input_nnz,
sketch_size, seed=None):
'''
input_rows: row indices for data (can be repeats)
input_data: values seen in row location,
input_nnz : number of nonzers in the data (can replace with
len(input_data) but avoided here for speed)
sketch_size: int
seed=None : random seed
'''
hashed_rows = np.empty(input_rows.shape,dtype=np.int32)
current_row = 0
hash_val = npr.choice(sketch_size)
sign_val = npr.choice(np.array([-1.0,1.0]))
#print(hash_val)
hashed_rows[0] = hash_val
#print(hash_val)
for idx in np.arange(input_nnz):
print(idx)
row_id = input_rows[idx]
data_val = input_data[idx]
if row_id == current_row:
hashed_rows[idx] = hash_val
input_data[idx] = sign_val*data_val
else:
# make new hashes
hash_val = npr.choice(sketch_size)
sign_val = npr.choice(np.array([-1.0,1.0]))
hashed_rows[idx] = hash_val
input_data[idx] = sign_val*data_val
return hashed_rows, input_data
def sort_row_order(input_data):
sorted_row_column = np.array((input_data.row,
input_data.col,
input_data.data))
idx = np.argsort(sorted_row_column[0])
sorted_rows = np.array(sorted_row_column[0,idx], dtype=np.int32)
sorted_cols = np.array(sorted_row_column[1,idx], dtype=np.int32)
sorted_data = np.array(sorted_row_column[2,idx], dtype=np.float64)
return sorted_rows, sorted_cols, sorted_data
if __name__=="__main__":
import time
from tabulate import tabulate
matrix = sparse.random(1000, 50, 0.1)
x = np.random.randn(matrix.shape[1])
true_norm = np.linalg.norm(matrix@x,ord=2)**2
tidy_data = sort_row_order(matrix)
sketch_size = 300
start = time.time()
hashed_rows, sketched_data = countSketch(tidy_data[0],
tidy_data[2], matrix.nnz,sketch_size)
duration_slow = time.time() - start
S_A = sparse.coo_matrix((sketched_data, (hashed_rows,matrix.col)))
approx_norm_slow = np.linalg.norm(S_A@x,ord=2)**2
rel_error_slow = approx_norm_slow/true_norm
#print("Sketch time: ".format(duration_slow))
start = time.time()
hashed_rows, sketched_data = countSketch(tidy_data[0],
tidy_data[2], matrix.nnz,sketch_size)
duration = time.time() - start
#print("Sketch time: ".format(duration))
S_A = sparse.coo_matrix((sketched_data, (hashed_rows,matrix.col)))
approx_norm = np.linalg.norm(S_A@x,ord=2)**2
rel_error = approx_norm/true_norm
#print("Relative norms: ".format(approx_norm/true_norm))
print(tabulate([[duration_slow, rel_error_slow, 'Yes'],
[duration, rel_error, 'No']],
headers=['Sketch Time', 'Relative Error', 'Dry Run'],
tablefmt='orgtbl'))
python algorithm numpy stream scipy
python algorithm numpy stream scipy
asked May 17 '18 at 21:24
Charlie DickensCharlie Dickens
1
1
bumped to the homepage by Community♦ 14 mins ago
This question has answers that may be good or bad; the system has marked it active so that they can be reviewed.
bumped to the homepage by Community♦ 14 mins ago
This question has answers that may be good or bad; the system has marked it active so that they can be reviewed.
$begingroup$
"... the latter is consistently too small_" does that mean it is not working correctly?
$endgroup$
– Sᴀᴍ Onᴇᴌᴀ
May 17 '18 at 21:46
add a comment |
$begingroup$
"... the latter is consistently too small_" does that mean it is not working correctly?
$endgroup$
– Sᴀᴍ Onᴇᴌᴀ
May 17 '18 at 21:46
$begingroup$
"... the latter is consistently too small_" does that mean it is not working correctly?
$endgroup$
– Sᴀᴍ Onᴇᴌᴀ
May 17 '18 at 21:46
$begingroup$
"... the latter is consistently too small_" does that mean it is not working correctly?
$endgroup$
– Sᴀᴍ Onᴇᴌᴀ
May 17 '18 at 21:46
add a comment |
1 Answer
1
active
oldest
votes
$begingroup$
as suggested by @SamOnela, code not working is off-topic. for your performance issue, you can group your calls to choice
at the beginning of your function
hash_vals = npr.choice(sketch_size, input_nnz)
sign_vals = npr.choice(np.array([-1.0,1.0]), input_nnz)
and use it later in your code this way:
hashed_rows[idx] = hash_vals[idx]
input_data[idx] = sign_vals[idx]*data_val
$endgroup$
add a comment |
Your Answer
StackExchange.ifUsing("editor", function ()
StackExchange.using("externalEditor", function ()
StackExchange.using("snippets", function ()
StackExchange.snippets.init();
);
);
, "code-snippets");
StackExchange.ready(function()
var channelOptions =
tags: "".split(" "),
id: "196"
;
initTagRenderer("".split(" "), "".split(" "), channelOptions);
StackExchange.using("externalEditor", function()
// Have to fire editor after snippets, if snippets enabled
if (StackExchange.settings.snippets.snippetsEnabled)
StackExchange.using("snippets", function()
createEditor();
);
else
createEditor();
);
function createEditor()
StackExchange.prepareEditor(
heartbeatType: 'answer',
autoActivateHeartbeat: false,
convertImagesToLinks: false,
noModals: true,
showLowRepImageUploadWarning: true,
reputationToPostImages: null,
bindNavPrevention: true,
postfix: "",
imageUploader:
brandingHtml: "Powered by u003ca class="icon-imgur-white" href="https://imgur.com/"u003eu003c/au003e",
contentPolicyHtml: "User contributions licensed under u003ca href="https://creativecommons.org/licenses/by-sa/3.0/"u003ecc by-sa 3.0 with attribution requiredu003c/au003e u003ca href="https://stackoverflow.com/legal/content-policy"u003e(content policy)u003c/au003e",
allowUrls: true
,
onDemand: true,
discardSelector: ".discard-answer"
,immediatelyShowMarkdownHelp:true
);
);
Sign up or log in
StackExchange.ready(function ()
StackExchange.helpers.onClickDraftSave('#login-link');
var $window = $(window),
onScroll = function(e)
var $elem = $('.new-login-left'),
docViewTop = $window.scrollTop(),
docViewBottom = docViewTop + $window.height(),
elemTop = $elem.offset().top,
elemBottom = elemTop + $elem.height();
if ((docViewTop elemBottom))
StackExchange.using('gps', function() StackExchange.gps.track('embedded_signup_form.view', location: 'question_page' ); );
$window.unbind('scroll', onScroll);
;
$window.on('scroll', onScroll);
);
Sign up using Google
Sign up using Facebook
Sign up using Email and Password
Post as a guest
Required, but never shown
StackExchange.ready(
function ()
StackExchange.openid.initPostLogin('.new-post-login', 'https%3a%2f%2fcodereview.stackexchange.com%2fquestions%2f194658%2fensuring-performance-of-sketching-streaming-algorithm-countsketch%23new-answer', 'question_page');
);
Post as a guest
Required, but never shown
1 Answer
1
active
oldest
votes
1 Answer
1
active
oldest
votes
active
oldest
votes
active
oldest
votes
$begingroup$
as suggested by @SamOnela, code not working is off-topic. for your performance issue, you can group your calls to choice
at the beginning of your function
hash_vals = npr.choice(sketch_size, input_nnz)
sign_vals = npr.choice(np.array([-1.0,1.0]), input_nnz)
and use it later in your code this way:
hashed_rows[idx] = hash_vals[idx]
input_data[idx] = sign_vals[idx]*data_val
$endgroup$
add a comment |
$begingroup$
as suggested by @SamOnela, code not working is off-topic. for your performance issue, you can group your calls to choice
at the beginning of your function
hash_vals = npr.choice(sketch_size, input_nnz)
sign_vals = npr.choice(np.array([-1.0,1.0]), input_nnz)
and use it later in your code this way:
hashed_rows[idx] = hash_vals[idx]
input_data[idx] = sign_vals[idx]*data_val
$endgroup$
add a comment |
$begingroup$
as suggested by @SamOnela, code not working is off-topic. for your performance issue, you can group your calls to choice
at the beginning of your function
hash_vals = npr.choice(sketch_size, input_nnz)
sign_vals = npr.choice(np.array([-1.0,1.0]), input_nnz)
and use it later in your code this way:
hashed_rows[idx] = hash_vals[idx]
input_data[idx] = sign_vals[idx]*data_val
$endgroup$
as suggested by @SamOnela, code not working is off-topic. for your performance issue, you can group your calls to choice
at the beginning of your function
hash_vals = npr.choice(sketch_size, input_nnz)
sign_vals = npr.choice(np.array([-1.0,1.0]), input_nnz)
and use it later in your code this way:
hashed_rows[idx] = hash_vals[idx]
input_data[idx] = sign_vals[idx]*data_val
edited May 18 '18 at 1:08
answered May 18 '18 at 0:15


bobrobbobbobrobbob
21215
21215
add a comment |
add a comment |
Thanks for contributing an answer to Code Review Stack Exchange!
- Please be sure to answer the question. Provide details and share your research!
But avoid …
- Asking for help, clarification, or responding to other answers.
- Making statements based on opinion; back them up with references or personal experience.
Use MathJax to format equations. MathJax reference.
To learn more, see our tips on writing great answers.
Sign up or log in
StackExchange.ready(function ()
StackExchange.helpers.onClickDraftSave('#login-link');
var $window = $(window),
onScroll = function(e)
var $elem = $('.new-login-left'),
docViewTop = $window.scrollTop(),
docViewBottom = docViewTop + $window.height(),
elemTop = $elem.offset().top,
elemBottom = elemTop + $elem.height();
if ((docViewTop elemBottom))
StackExchange.using('gps', function() StackExchange.gps.track('embedded_signup_form.view', location: 'question_page' ); );
$window.unbind('scroll', onScroll);
;
$window.on('scroll', onScroll);
);
Sign up using Google
Sign up using Facebook
Sign up using Email and Password
Post as a guest
Required, but never shown
StackExchange.ready(
function ()
StackExchange.openid.initPostLogin('.new-post-login', 'https%3a%2f%2fcodereview.stackexchange.com%2fquestions%2f194658%2fensuring-performance-of-sketching-streaming-algorithm-countsketch%23new-answer', 'question_page');
);
Post as a guest
Required, but never shown
Sign up or log in
StackExchange.ready(function ()
StackExchange.helpers.onClickDraftSave('#login-link');
var $window = $(window),
onScroll = function(e)
var $elem = $('.new-login-left'),
docViewTop = $window.scrollTop(),
docViewBottom = docViewTop + $window.height(),
elemTop = $elem.offset().top,
elemBottom = elemTop + $elem.height();
if ((docViewTop elemBottom))
StackExchange.using('gps', function() StackExchange.gps.track('embedded_signup_form.view', location: 'question_page' ); );
$window.unbind('scroll', onScroll);
;
$window.on('scroll', onScroll);
);
Sign up using Google
Sign up using Facebook
Sign up using Email and Password
Post as a guest
Required, but never shown
Sign up or log in
StackExchange.ready(function ()
StackExchange.helpers.onClickDraftSave('#login-link');
var $window = $(window),
onScroll = function(e)
var $elem = $('.new-login-left'),
docViewTop = $window.scrollTop(),
docViewBottom = docViewTop + $window.height(),
elemTop = $elem.offset().top,
elemBottom = elemTop + $elem.height();
if ((docViewTop elemBottom))
StackExchange.using('gps', function() StackExchange.gps.track('embedded_signup_form.view', location: 'question_page' ); );
$window.unbind('scroll', onScroll);
;
$window.on('scroll', onScroll);
);
Sign up using Google
Sign up using Facebook
Sign up using Email and Password
Post as a guest
Required, but never shown
Sign up or log in
StackExchange.ready(function ()
StackExchange.helpers.onClickDraftSave('#login-link');
var $window = $(window),
onScroll = function(e)
var $elem = $('.new-login-left'),
docViewTop = $window.scrollTop(),
docViewBottom = docViewTop + $window.height(),
elemTop = $elem.offset().top,
elemBottom = elemTop + $elem.height();
if ((docViewTop elemBottom))
StackExchange.using('gps', function() StackExchange.gps.track('embedded_signup_form.view', location: 'question_page' ); );
$window.unbind('scroll', onScroll);
;
$window.on('scroll', onScroll);
);
Sign up using Google
Sign up using Facebook
Sign up using Email and Password
Sign up using Google
Sign up using Facebook
Sign up using Email and Password
Post as a guest
Required, but never shown
Required, but never shown
Required, but never shown
Required, but never shown
Required, but never shown
Required, but never shown
Required, but never shown
Required, but never shown
Required, but never shown
tTNsDt,fJ59lrYb,1GGts ra03eIvrTp rep0xm
$begingroup$
"... the latter is consistently too small_" does that mean it is not working correctly?
$endgroup$
– Sᴀᴍ Onᴇᴌᴀ
May 17 '18 at 21:46